15: Quantitative Reasoning and Information Literacy in Economics
- Page ID
- 70140
Chapter 15. Quantitative Reasoning and Information Literacy in Economics
Illinois Wesleyan University
Introduction
The Association of College and Research Libraries’ (ACRL) Framework for Information Literacy for Higher Education (Framework for IL) (2015) defines information literacy (IL) as a “spectrum of abilities, practices, and habits of mind.” Articulating IL as a framework—as opposed to a set of standards or learning outcomes—ACRL articulates six different frames. Each frame identifies a threshold concept central to IL and includes learner-based recommended knowledge practices (“demonstrations of understanding”) and aspirational dispositions (e.g., attitudes and values). As ACRL emphasizes “flexible options for implementation” of the Framework for IL, it effectively outlines an open-ended learning process while providing abundant reference checkpoints.
My 17 years of teaching undergraduate economics has led me to interpret and articulate ACRL’s Framework for IL along pedagogical lines that closely overlap several of the literacy categories created by Jeremy J. Shapiro and Shelley K. Hughes (1996) as well as the streamlined IL process outlined by Tom W. Goad (2002). In addition, the central role that statistical data plays in the discipline of economics makes the concept of quantitative literacy, or numeracy, very relevant for our students. The National Numeracy Network (NNN) (2015) defines quantitative literacy as the “comfort, competency, and ‘habit of mind’ in working with numerical data,” and the skill of IL is a critical means to achieve this kind of numeracy in economics.
Three frames in the Framework for IL are central to the education of economics majors: Searching Is Strategic emphasizes research as a process; Information Has Value underlines the social and historical context of information; and Research as Inquiry highlights the management and synthesis of information. ACRL (2009) references the work of Shapiro and Hughes (1996) in order to communicate the “substance and breadth of information literacy” to faculty and administrators, and I find it useful to borrow their language in order to relate the Framework for IL to the undergraduate instruction of economics. Shapiro and Hughes list a series of desirable characteristics in an IL curriculum, namely: tool literacy, resource literacy, social-structural literacy, research literacy, publishing literacy, emerging technology literacy, and critical literacy.
Given that the ACRL Instruction Section Information Literacy in the Disciplines Committee (ACRL IS) (2012) reports that it “is not aware of information literacy standards in this area,” I propose adopting the dimensions of literacy put forward by Shapiro and Hughes (1996). Desirable as they all are in an “enlightened” liberal arts curriculum, three are particularly relevant in the context of the social sciences curriculum in general and of that of economics in particular. Describing resource literacy as “the ability to understand the form, format, location and access methods of information resources,” these authors speak to the use of both text/qualitative information and data/quantitative information that students and researchers must employ in their work. Closely related to this dimension of literacy is that of social-structural literacy, described as “knowing that and how information is socially situated and produced.” The difference between for-pay (i.e., proprietary) and public-access information, for example, becomes critical when attempting to operationalize a research project in economics. Finally, research literacy, described as “the ability to understand and use the Information Technology (IT)-based tools relevant to the work of today’s researcher and scholar” specifically references “computer software for quantitative analysis.” At the undergraduate educational level this quantitative analysis is frequently performed through the use of spreadsheets.
These particular dimensions of literacy underpin the “information literacy strategy” outlined by Goad (2002, p. 36) through a series of sequential tasks. These are: (i) formulating a question, (ii) pinpointing what you really want to know, (iii) organizing information, (iv) planning a search for relevant information, and (v) evaluating the appropriateness of materials. As the work of Goad (2002) fully articulates the connection between IL and workplace performance, I will argue that the multi-step information search and analysis process that he describes closely overlaps several of the desirable learning goals of the economics curriculum. Encapsulated in the phrase coined by John J. Siegfried et al. (1991) “thinking like an economist,” these goals include “acquiring and using knowledge that cuts across disciplinary boundaries” and include–among others–the threshold concepts of “knowing something about the measurement of economic variables (methods of data collection, reliability, etc.)” and “being able to organize, work with, and manipulate data for purposes of comparison” (Siegfried et al., 1991, p. 216). Moreover, “the use of analytical methods to utilize information” is identified by Dale Cyphert and Stanley P. Lyle (Chapter 3, this collection) as one of the skill gaps of greatest concern to employers. For an in-depth discussion of the expected proficiencies of the economics academic major I direct the reader to the seminal work of W. Lee Hansen (1986). His influence in shaping the contemporary effort to “educate economists” is explicitly stated in the collected works edited by David Colander and KimMarie McGoldrick (2009). In sum, the instructional challenge lies in designing course assignments that help students develop the aforementioned intellectual proficiencies, relating numeracy to information literacy.
The pedagogical approach that I propose is based on the educational taxonomy originally proposed by Benjamin Bloom (1956) and it employs case method teaching in an intermediate macroeconomic theory course. The work of Lorin W. Anderson and Lauren A. Sosniak (1994) provides a helpful 40-year retrospective on the impact of Bloom’s taxonomy on a wide-range of pedagogical issues and practices. Along those lines, I will posit that the use of quantitative case studies as a pedagogical resource in intermediate macroeconomics helps students analyze and evaluate theoretical constructs in economics. Specifically, the collection, manipulation and analysis of data compiled by different statistical agencies illustrate for students the connection between the theoretical and empirical dimensions of this particular social science. I believe that the process of building such a connection relies heavily on the parallel development of a basic set of IL skills.
From the course instructor’s perspective, the overall goal of the proposed pedagogical strategy is to move students from Bloom’s (1956) lower-order cognitive processes of knowledge, comprehension and application of intermediate macroeconomic abstract formal thinking to the higher-order cognitive processes of analysis, synthesis and evaluation of this mode of thinking. In the discipline of economics, as in almost all social sciences, the analysis and evaluation of theoretical constructs are based upon the statistical manipulation of data. In economics, these data are generally quantitative (rather than qualitative) and, depending on the topic of study, may have been generated by the researcher (e.g., surveys) or collected from public agencies such as statistical agencies. In the sub-field of macroeconomics all the data are generated and collected by public agencies.
In order to bridge the cognitive gap between the discussion of theoretical concepts and the manipulation and evaluation of these concepts I propose to borrow from the case method teaching pedagogy. As described by Melvin Copeland (1954), it was originally created in a business and management learning environment whereas, nowadays, Geoff Easton (1983) and James A. Erskin, Michiel R. Leenders, and Louise A. Mauffette-Leenders (1998) show the case method spread to many other disciplines. In essence, cases are context-rich real world stories that students, usually working in groups, analyze in order to answer a question or solve a problem. These cases provide students with quantitative information but unlike problem sets or examples they do not have a unique “correct” answer. The case method teaching pedagogy has three main components: the case itself, the students’ preparation for the case, and the discussion that takes place in the classroom. It is the collaborative nature, both outside and inside of the classroom, of this evidence-based exercise that improves the grasp of theoretical concepts and their application to real-world situations. Katt Blackwell-Starnes (Chapter 7, this collection), provides a discussion of course assignment design in relation to IL. Blackwell-Starnes’ study of IL skills applied to undergraduate research leads her to endorse assignment design. In the course activity that I describe in the following sections, students apply a series of economic concepts to sketch the actual macroeconomic profile of a country.
Although many teaching faculty members will argue that there is “no room in the syllabus” for the inclusion of explicit IL goals in a standard course in intermediate macroeconomic theory I envision—as discussed above—the proposed pedagogical strategy to be closely aligned with several frames in the Framework for IL. Later on in their academic and professional careers, when students develop independent research projects showcasing their “thinking like an economist” skills, they are likely to be savvier gatherers and users of information.
The work of Pam McKinney (2013) summarizing the lessons learned from a multi-year, multi-disciplinary curriculum development effort gives credence to the usefulness of “inquiry-based” learning to the development of IL skills. For a complete discussion of how to teach IL for inquiry-based learning I direct the reader to Mark Hepworth and Geoff Walton (2009). By presenting students with a series of questions to answer and argue, the quantitative case study method that I propose exposes students to what McKinney describes as “collaborative inquiry.” The kind of longitudinal data that she analyzes offers evidence of how inquiry-based learning increases student appreciation of IL competencies. Unfortunately, I currently lack her ability to track student skills as they move through our curriculum.
In what follows, I will outline the challenge that motivates my particular pedagogical approach, assess its impact on exam performance, and relate its implementation to the development of numeracy, resource literacy, social-structural literacy, and research literacy among students of Intermediate Macroeconomics. This research was approved by the Institutional Review Board at Illinois Wesleyan University on December 3rd, 2012.
The Problem
In the course sequence for a minor or a major in economics, students at my institution start with an Introduction to Economics (ECON 100) course. Frequently, the content of this course is split into two separate courses, Introduction to Microeconomics and Introduction to Macroeconomics, which exposes students to a wide spectrum of microeconomic and macroeconomic concepts. Whether in a single course or in a two-course sequence the purpose of this introduction is widely acknowledged to be the initiation of students into what is commonly referred to as “economic thinking”: an analytical comparison of costs and benefits of different choices regarding the allocation of scarce resources. This course is mostly devoid of mathematics and only makes superficial use of abstract thinking through algebra. Graphical analysis is limited to supply and demand diagrams and to the plotting of time series data in order to illustrate either trends or relative values.
After completing the introductory course, a student at my institution interested in economics as a major field of study is required to complete two courses covering intermediate-level economic theory: Intermediate Microeconomics (ECON 201) and Intermediate Macroeconomics (ECON 202). As outlined by David Colander and KimMarie McGoldrick (2009), in these units of the standard disciplinary curriculum the focus turns to the theories and identities underpinning the basic concepts discussed in the introductory course (p. 29-30). Two examples would serve to illustrate this point. The introductory discussion of economic growth is now enriched through the articulation of the Solow growth model where Robert Solow (1956) provides a theoretical model of economic growth that has become the basic framework for research since. Also, the interplay between nominal and real variables is now presented through the stylized fact of the Phillips Curve where William A. Phillips (1958) argues that there is a secular negative relationship between the inflation rate (a nominal variable) and the unemployment rate (a real variable). His work was subsequently replicated by Milton Friedman (1968) and Edmund Phelps (1970) and sparked a fierce intellectual debate in the discipline. (For a summary of both economic concepts, please see the Palgrave Dictionary of Economics online.) In order to develop these theoretical concepts an Intermediate Macroeconomics course makes frequent use of algebra–for purposes of manipulating concepts in symbolic form–and may even employ calculus to derive the different equations that compose the Solow growth model. Graphical analysis is also more complex, employing diagrams with multiple lines and curves intersecting at different points, as well as plotting time series data in order to illustrate cycles. For a discussion of statistical data visualization pedagogy I direct the reader to my article on data visualization and the FRED database (Méndez-Carbajo, 2015).
My experience has been that students tend to struggle when confronted with the sequential tasks of learning the economic theories and concepts, applying these theories and concepts to specific problems, and using evidence to discuss the limitations of the theories and the applicability of the concepts. The work of Ann D. Velenchik (1995) discusses these issues in detail. The aforementioned learning tasks are more demanding of our students than those they were faced with in ECON 100, not only because they employ more formal mathematical analysis but also because their application is more fluid and less clear-cut. For example, the discussion of historical shifts of the Phillips Curve requires referencing a historical context usually external to the course content and potentially controversial in and of itself. In addition, students are faced with the need to develop a new vocabulary, that of quantitative information. Continuing with the example of the Phillips Curve, students learn about how U.S. inflation increased three-fold in the 1970s and are expected to compare that figure with a doubling of the unemployment rate during the same period. In other words, students need to learn to evaluate issues of magnitude and proportion.
Finally, there is, the challenge of “thinking in macroeconomic terms”–as opposed to “thinking in microeconomic terms.” In an introductory course we as instructors undertake considerable efforts to make the course material relevant and engaging through the use of examples and illustrations close to the students’ “micro” reality, for example, discussing opportunity cost in terms of hours of sleep versus hours of study. Because students are familiar with these kinds of information they tend to find “thinking in microeconomic terms” rather easy and, to an extent, intuitive. At the same time, I would argue there is a large information deficit when it comes to the “macro” reality that the students live in. In my experience, beginning-of-the-semester student surveys on current inflation or GDP growth rates reveal great gaps in students’ familiarity with macroeconomic information. I would argue that this degree of information ignorance makes the task of “thinking in macroeconomic terms” more difficult. In the detailed introduction to their book, Colander and McGoldrick (2009) discuss the traditional lack of “context” in the teaching of intermediate macroeconomic theory. Describing this information deficit as pervasive they argue for improved pedagogical practices that “enhance the use of context and application” (Colander & McGoldrick, 2009, p. 33)
The Activity
The ECON 202 course where I implemented this pedagogical innovation is organized around four units of content: (1) Introduction (4 class periods), (2) Long-Run Economic Performance (6 class periods), (3) Business Cycles and Macroeconomic Policy (8 class periods), and (4) Macroeconomic Policy (6 class periods). There are bi-weekly online quizzes and two in-class partial exams, week 6 and week 12, as well as a comprehensive final exam, week 16. As a voluntary activity for extra credit, during the last week of classes students can deliver a group presentation on the macroeconomic conditions of the country that they are assigned to at the beginning of the semester.
During the first class period of the semester the students meet the academic librarian who serves as the liaison with the Economics Department for a research instruction session at the library’s computer lab. There, the students are introduced to the database that they will use to gather the data for the quantitative case studies and are assigned to one of four different work groups. The academic librarian focuses on the area of resource IL, discussing with the students the means of access, the forms, and the formats of quantitative information relevant to this course. At this point, the Framework for IL’s “Searching Is Strategic” frame is most prominently highlighted. This research instruction session also serves to introduce the academic librarian to the students in order to encourage them to seek her/his assistance with database needs throughout the semester. See also Alison S. Gregory and Betty L. McCall’s (Chapter 18, this collection) discussion of a teaching faculty/librarian collaborative approach to teaching IL skills in the context of a sociology capstone course.
The library subscribes to the International Financial Statistics (IFS) online database maintained by the International Monetary Fund (IMF), and I have found this resource very convenient for the purposes of my course. In order to keep the data analysis and the size of the work groups manageable each semester I identify four or five different countries for the students to study. I purposely select countries at varied stages of economic development in order to highlight socio-structural differences in economic performance. During the research instruction session at the library all students work on gathering data on the GDP components for the U.S., also becoming familiar with the online teaching platform Moodle, locating the discussion questions for the quantitative case studies, and importing their data into Microsoft Excel for purposes of analysis. This first quantitative case study, and all that follow, is structured around a one-page handout that I distribute in class. Table 1 in Appendix A contains a list of the topics of study, the variables that students extract from the database, the manipulations that students must perform on the series, and the discussion questions that they need to answer based on the graphical representation of their data. I will discuss these tasks in sequence.
Currently, I have incorporated a quantitative case study component for each of the following seven topics in a standard intermediate macroeconomics course: (1) GDP components, (2) Uses of Saving, (3) Productivity, (4) Growth, (5) Inflation, (6) Interest Rates, and (7) the Phillips Curve. Each of these concepts and topics are first presented from a conventional theoretical perspective and immediately compared against their historical record in the United States. I then direct students to compile, after class, the relevant data from the IFS database and to plot those data into Microsoft Excel spreadsheets. Figure 15.1 presents a sample of those plots.
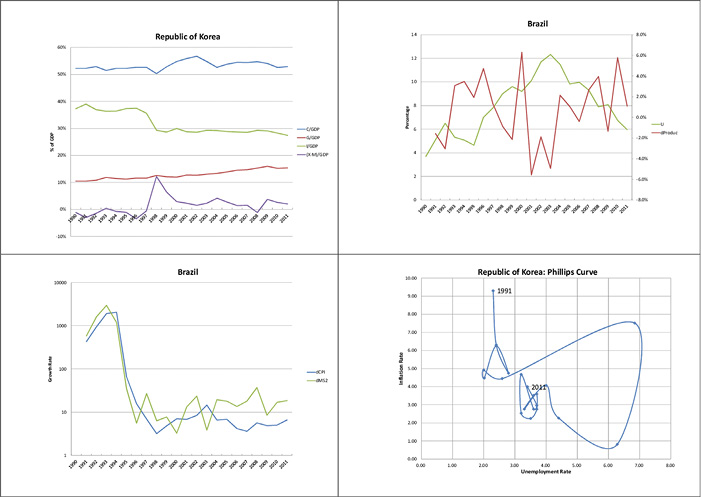
Figure 15.1. Sample data plots
Depending on the topic, students are asked to compute ratios between variables (topic 1), rates of growth (topics 2, 3, and 5), sums or subtractions (topics 2, 4 and 6), or to generate a scatter plot (topic 7). The use of spreadsheets for data manipulation and plotting is common practice in the discipline and this element of the activity contributes to the development of research literacy skills. At this point, the Framework for IL’s Research as Inquiry frame is most prominently highlighted. Also, it is usually at this stage when students begin to be aware of matters germane to social-structural IL. For example, changes in the methodology of data collection, or even a change in the definition of the object of study (e.g., Germany pre-and-post 1990), result in discontinuities in the series–or even gaps. In a similar light, time periods when the variables are very large in magnitude (e.g., Brazil’s hyper-inflation in the 1990s) dwarf the rest of the data, making their visual interpretation much more difficult. Although these data-related issues are sometimes frustrating to some students, they are excellent educational opportunities to develop a historical context to the study of macroeconomics.
After the data is plotted, students use the graphs they have generated to answer a series of discussion questions highlighting how quantitative evidence validates—and sometimes challenges—the theoretical relationships focus of the course. Table 2 in Appendix A contains the list of discussion questions per topic. These questions are posted on an online discussion forum hosted on the Moodle teaching platform. Only students registered in the course have access to these questions and the forum is set up in a “Q&A” format, which prevents individual students from seeing their peers’ answers to the common set of questions until they have posted their own work. Students have no less than 48 hours to complete the data collection, plotting, and analysis before the submission of their answers is blocked. In other words, their work must be completed and uploaded to Moodle by the time the class convenes the following day. The discussion questions associated with each quantitative case study cover a range of issues. Some questions ask the students to describe visual aspects of the data (e.g., “which GDP component is the largest?”) and aim to be prompts for discussion of economic structures when different countries’ data are compared. Some questions (e.g., “Is the country a net lender or a net borrower?”) require the application of theoretical concepts discussed in class to the changing reality of different economies. Finally, some other questions (e.g., “Do the growth rate of labor productivity and the unemployment rate move in the same direction? Why not?”) aim to bring to the fore the theoretical relationships object of the course.
During the class period when the quantitative case studies are discussed the students’ work—both their data plots and their answer to the discussion questions—are projected on video screens. Over the last three semesters I have made use of a technology-intensive classroom setup with multiple video projectors and a digital whiteboard. Those are not essential components of this activity but they facilitate the visualization of trends, cycles, and degrees of association between variables. Moreover, I believe the fact that students see their work projected for everybody else to see serves to produce a certain degree of peer pressure that marginally improves the average quality of their work. It is during the class period when the case studies are discussed that quantitative literacy, or numeracy, becomes central to the course. As the students work through their individual discussion questions they gain confidence in reading and interpreting the data associated with the assignment. Also, as the same discussion question is addressed across different countries students are able to observe different orders of magnitude, proportion, and signs of macroeconomic magnitudes under study, effectively developing a true context for their theoretical study of economics. At this point, Framework for IL’s Information Has Value frame is most prominently highlighted.
Assessment
For the purpose of this chapter I will focus on the challenges associated with the discussion of a standard production function, the derivation of the concept of labor productivity, its connection to the demand for labor, and, finally, to the concept of the unemployment rate. This is the quantitative case study number 3 summarized in Table 1 in Appendix A.
Over the years, most of my in-class exams have included questions on these concepts. The phrasing of the questions has evolved but the focus remains on the same issue: economic theory teaches us that as productivity increases, other things being equal, the unemployment rate decreases. In my experience, students tend to struggle with the notion that as workers become more productive the demand for their labor increases. Their “micro” thinking, discussed earlier in this chapter, leads them to conclude that employers demand fewer workers once these workers become more productive.
In the fall semesters of 2008 and 2009, prior to the inclusion of quantitative case studies in the course, I asked students to identify in a diagram the impact of an increase in total factor productivity on output and on the marginal productivity of labor. Although, in general terms, the students displayed their knowledge of the concepts at stake through a proficient replication of the graphs discussed in class, they struggled when confronted with the task of evaluating a reporter’s statement contradicting intermediate macroeconomic theory. In fact, as the students were asked to analyze information, rather than to replicate material covered in lectures, the average scores on these specific questions dropped from 75% to 34% and their standard deviations increased from 0.15 to 0.25 with population sizes (N) of 8 in both semesters. Due to the small size of the populations under study I will not attempt to draw conclusions about the statistical robustness of these figures. Nevertheless, one could argue that as students were pushed up the skill pyramid representing Bloom’s (1956) taxonomy of learning objectives they faltered at the more demanding task.
In the fall semesters of 2011 and 2012, after the inclusion of quantitative case studies in the course, I asked students to identify in the same sets of diagrams the impact of decreases in either capital expenditure or in total factor productivity on output, the marginal productivity of labor, and the unemployment rate. The phrasing of the questions was more specific than in previous tests but it also demanded that students relate changes in production and in labor productivity to changes in the unemployment rate. The average scores on these questions were 57% in 2011 and 62% in 2012, with associated standard deviations of 0.41 and 0.29. Population sizes (N) were 14 and 17, respectively. Notice that although the standard deviations of the post-quantitative case study test scores have increased relative to the pre-quantitative case study test scores, the 2009 and 2012 values are very similar.
Student reflections on the use of quantitative case studies have been overwhelmingly positive. Quoting from the university’s standard anonymous course evaluations, students state that: “I feel using real data to help support economic theories was extremely useful,” “I also liked the discussion questions because they helped apply and reinforce ideas,” “Learning how to analyze graphs and data and how to properly interpret that data were valuable skills to learn,” and “I feel like putting the effort to read notes and work on the IMF forums [i.e., the discussion questions on the IFS-IMF data-based quantitative case studies] paid off and led me to learn/understand more about macroeconomics.” In the form of suggestions, some students state their desire for “more in-class activities or assignments along the way” or notice how the course was “more lecture-oriented rather than discussion-oriented.” I believe that at this point in time it would be very difficult to introduce more of these activities without substantially impacting the primary goal of the course (i.e., the mastering of intermediate macroeconomic theory). Having said that, the benefits of addressing Framework for IL threshold concepts through this particular pedagogical strategy are observable through the student’s appreciation of the intellectual tasks of “understanding” rather than memorizing, “interpreting” rather than mimicking, and “analyzing” rather than replicating.
Discussion
The goal of introducing elements of case method teaching into an intermediate macroeconomic theory course has been to make student thinking more sophisticated and context-rich. The design of activities where students collect, manipulate, and analyze data also contributes to develop critical IL skills. Replacing many of the exercises aimed at rote replication of the content of lectures, the activities organized around quantitative case studies require from students a more extended and sophisticated engagement with the material. Thus, I will argue, students more effectively apply theories and concepts to specific problems and are more capable at using quantitative evidence to discuss the limitations of the economic theories. Moreover, the nature of the case method and its use of real data allow the instructor to present students with the fact that macroeconomic concepts and theories are frequently dynamic and thus mutable. Finally, I believe that the continued exposure to macroeconomic data, their sources, and even orders of magnitude, builds a framework of reference for students that help them start to develop the desirable quality of “thinking in macroeconomic terms.”
Although it is not listed as an explicit learning goal of the course I consider the described quantitative case studies a solid pedagogical strategy in the education of my students in matters of IL in the discipline: by providing instruction on the form, format, location, and access of quantitative data as a central part of the course assignments my students develop resource literacy; by discussing the process of creating and organizing statistical information and knowledge across countries and across time my students develop socio-structural literacy; and by understanding and using spreadsheets to perform quantitative analysis of the statistical information that they have collected my students develop research literacy. These literacy skills are not presented as ends by themselves, yet they become—in my opinion—critical means to develop quantitative literacy, or numeracy, among the students. Finally, as the students locate and use effectively the quantitative information that they need to evaluate intermediate macroeconomic concepts, they are in effect developing the connection between theories and empirical evidence that underpins the social science of economics.
In future iterations of the course I will consider highlighting explicit issues of social-structural literacy, creating discussion questions specific to the social situation and production of the data of each assignment. These questions may ask students to identify the agency compiling the data, the highest frequency that the data are available, and potential reasons for changes in collection methodology. By doing so I hope to draw student attention to the fact that social groups create and organize information as I continue to teach the students how to manipulate and interpret that information. Also looking forward, I hope to develop, in collaboration with the academic librarian liaison to the Department and as part of the multi-year assessment effort of our student learning goals, a rubric for IL outcomes in our program. Since we already enjoy a long history of collaboration in the delivery of instructional sessions on locating, evaluating, and using text/qualitative information as part of the capstone (i.e., senior-level) course, I believe our academic library liaison to be a key partner in this effort. As a first step I will suggest borrowing from the ACRL-guided work in other social sciences, such as political science, to develop a research competency guide for our discipline.
References
Association of College & Research Libraries (ACRL). (2009). Information literacy for faculty and administrators. Retrieved from www.ala.org/acrl/issues/infol...aculty/faculty
Association of College & Research Libraries Instruction Section (ACRL IS). (2012). Information literacy in economics. Retrieved from wikis.ala.org/acrl/index.php/...y_in_Economics
Anderson, L. W., & Sosniak L. A. (Eds.). (1994). Bloom’s taxonomy: A forty-year retrospective. Chicago National Society for the Study of Education. Chicago, IL: University of Chicago Press.
Blackwell-Starnes, K. (2016). Preliminary paths to information literacy: Introducing research in core courses. In B. J. D’Angelo, S. Jamieson, B. Maid, & J. R. Walker (Eds.), Information literacy: Research and collaboration across disciplines. Fort Collins, CO: WAC Clearinghouse and University Press of Colorado.
Bloom, B. (1956). Taxonomy of educational objectives: the classification of educational goals. United Kingdom: Longman.
Colander, D., & McGoldrick, K. M. (Eds.). (2009). Educating economists: The Teagle discussion on re-evaluating the undergraduate economics major. Northampton, MA: Edward Elgar.
Copeland, M. (1954). The genesis of the case method in business instruction. In M. McNair & A. Hersum (Eds.), The case method at the Harvard Business School (pp. 25-33). New York, NY: McGraw-Hill.
Cyphert, D., & Lyle, S. P. (2016). Employer expectations of information literacy: Identifying the skills gap. In B. J. D’Angelo, S. Jamieson, B. Maid, & J. R. Walker (Eds.), Information literacy: Research and collaboration across disciplines. Fort Collins, CO: WAC Clearinghouse and University Press of Colorado.
Easton, G. (1983). Learning from case studies. London: Prentice Hall.
Erskine, J., Leenders, M., & Mauffette-Leenders, L. (1998). Teaching with cases. London, Ontario: Ivey Publishing.
Friedman, M. (1968). The role of monetary policy. American Economic Review, 58(1), 1-17.
Goad, T. (2002). Information literacy and workplace performance. Wesport, CT. Quorum Books.
Gregory, A. S., & McCall, B. L. (2016). Building critical researchers and writers incrementally: Vital partnerships between faculty and librarians. In B. J. D’Angelo, S. Jamieson, B. Maid, & J. R. Walker (Eds.), Information literacy: Research and collaboration across disciplines. Fort Collins, CO: WAC Clearinghouse and University Press of Colorado.
Hansen, L. (1986). What knowledge is most worth knowing for economics majors? American Economic Review, 76(2), 149-152.
Hepworth, M., & Walton, G. (2009). Teaching information literacy for inquiry-based learning. Oxford: Chandos Publishing.
McKinney, P. (2013). Information literacy and inquiry-based learning: Evaluation of a five-year programme of curriculum development. Journal of Librarianship and Information Science. Published online before printing: http://lis.sagepub.com/content/early...613477677.full
Méndez-Carbajo, D. (2015). Data visualization and the FRED database. Journal of Economic Education, 46(4), 420-429.
National Numeracy Network (2015, April 22). What is numeracy/QL/QR? Retrieved from http://serc.carleton.edu/nnn/resources/index.html
Phelps, E. (1970). Money wage dynamics and labor market equilibrium. In E. Phelps (Ed.), Microeconomic foundations of employment and inflation theory (pp. 124-166). New York., NY: W.W. Norton.
Phillips, W. A. (1958). The relation between unemployment and the rate of change of money wages in the United Kingdom, 1861-1957. Economica, 25(100), 283-299.
Shapiro, J. J., & Hughes, S. K. (1996). Information literacy as a liberal art: Enlightenment proposals for a new curriculum. Educom Review, 31(2).
Siegfried, J. J., Bartlett, R. L., Hansen, W. L., Kelley, A.C., McCloskey, D. N., & Tietenberg, T. H. (1991). The status and prospects of the economics major. Journal of Economic Education, 22(3), 197-224.
Solow, R. M. (1956). A contribution to the theory of economic growth. Quarterly Journal of Economics, 70(1), 65-94.
Velenchik, A. (1995). The case method as a strategy for teaching policy analysis to undergraduates. Journal of Economic Education, 26(1), pp. 29–38.
Appendix A
Topics of Study, Variables, Manipulation, and Discussion Questions
Table 1. GDP components
Concept |
Variable Name |
Unit |
C |
Private Final Consumption Expend., Nominal |
National Currency |
G |
Public Final Consumption Expend., Nominal |
National Currency |
I |
Gross Capital Formation, Nominal |
National Currency |
X |
Exports of Goods and Services, Nominal |
National Currency |
M |
Imports of Goods and Services, Nominal (-) |
National Currency |
GDP |
Gross Domestic Product (GDP) |
National Currency |
Computing a Ratio: (e.g. C / GDP)
New column = Column with C / Column with GDP
Number format is %
Discussion Questions:
- Which GDP component is the largest? Which is the smallest?
- Is there a trend in their evolution over time? Does this trend change direction?
- Are there any noticeable peaks or troughs that you can identify?
- Do net exports (NX=Exports-Imports) add or detract from overall GDP?
- What events could have caused specific ups and downs in the series?
Table 2. Uses of saving
Concept |
Variable Name |
Unit |
CA |
Current Account, Income, Credit |
US Dollars |
I |
Corp., Househ., and NPISH, Gross Fixed Capital Formation, Nominal |
National Currency |
ER |
National Currency per U.S. Dollar, per. aver. |
National Currency per US Dollar |
Computing a Sum or a Difference: (e.g. I + CA)
New column = Column with I + Column with CA
Discussion Questions:
- Is there a trend in the series? What does such a trend mean in terms of economic growth?
- Is there a noticeable cycle in the series? What could have created such a cycle?
- Do I and CA have the same sign? Do they move in the same direction?
- Do I and S have the same sign? Is one larger/smaller than the other?
- Is the country a net borrower or a net lender?
Table 3. Productivity
Concept |
Variable Name |
Unit |
EMP |
Employment |
Thousands |
UMP |
Unemployment Rate |
Percentage |
GDP |
Nominal Gross Domestic Product (GDP) |
Billions National Currency |
GDEF |
Gross Domestic Product Deflator |
Index Number |
Transforming into Real Values
New column (rGDP) = Column with Nominal GDP / (Column with GDP deflator / 100)
Computing a Growth Rate
Growth rate (in %) = [(New value – Old value) / Old Value] * 100
New column (dProduc) = % growth rate of Column with rGDP/Emp
Number format is %
Discussion Questions:
- Which of the two series is more volatile: the growth rate of labor productivity or the unemployment rate?
- Can you identify one (or several) cycles in the series? For what dates?
- Do the growth rate of labor productivity and the unemployment rate move in the same direction? Why not?
- Do they move “at the same time”? Does one “lead” the other? Why?
- What can you infer about overall economic activity based on the evolution of the unemployment rate?
Table 4. Interest rates
Concept |
Variable Name |
Unit |
CPI |
Consumer Price Index |
Index Number |
DR |
Bank/Discount rate (or Fed Funds rate) |
Percentage |
MMR |
Deposit rate (passive) |
Percentage |
LR |
Lending rate (lending) |
Percentage |
Computing a Growth Rate: (e.g. Rate of Inflation)
Growth rate (in %) = [(New value – Old value) / Old Value] * 100
Number format is %
Computing a Real Rate of Return: (e.g. Real Interest Rate)
Real interest rate = Nominal (reported) bank rate – Inflation rate (dCPI)
Discussion Questions:
- Is there a trend in the evolution of real interest rates over time?
- Are there cycles in the evolution of real interest rates over time?
- Which real interest rate is highest? Which is lowest? Why?
- What does it mean for a real interest rate to be negative?
- What does it mean for the financial system when the real deposit rate and the real lending rate are almost identical?
Table 5. Growth
Concept |
Variable Name |
Unit |
GDP |
Gross Domestic Product (GDP) |
National Currency |
GDEF |
Gross Domestic Product Deflator |
Index Number |
EMP |
Employment |
Thousands |
POP |
Population |
Millions |
Computing the Real GDP per capita: (i.e., Real GDP / Population)
New column (rGDPcap) = Column with Real GDP / Column with Population
Computing the growth rate of real GDP per capita
New column (drGDPcap) = % growth rate of Column with rGDPcap
Number format is %
Discussion Questions:
- Which of the two series is more volatile: the growth rate of labor productivity or the growth rate of per capita GDP?
- Can you identify any trends or cycles in the series? For what dates?
- Do the growth rate of labor productivity and the growth rate of per capita GDP move in the same direction? Why?
- Do they move “at the same time”? Does one “lead” the other? Why?
- Consider how labor productivity is calculated (i.e., rGDP/EMP). How can you explain spikes in its value (i.e., large increases in its growth rate)?
Table 6. Inflation
Concept |
Variable Name |
Unit |
CPI |
Consumer Price Index |
Index Number |
MS2 |
Money Supply: Aggregate #2 |
Billions of local currency |
Computing a Growth Rate: (e.g. Rate of Inflation)
Growth rate (in %) = [(New value – Old value) / Old Value] * 100
Discussion Questions:
- Is there a trend in the evolution of these variables over time?
- Are there any significant ups and downs?
- Do the rate of growth of money supplied and the rate of growth of prices move in sync?
- What policy factors affect M2 growth?
- What “real” factors affect M2 growth?
Table 7. Phillips Curve
Concept |
Variable Name |
Unit |
CPI |
Consumer Price Index |
Index Number |
U |
Unemployment Rate |
Percentage |
Computing a Growth Rate: (e.g. Rate of Inflation)
Growth rate (in %) = [(New value – Old value) / Old Value] * 100
Discussion Questions:
- What is, generally speaking, the slope of the spaghetti line connecting all the data pairs?
- For which years does the concept of the short-term Phillips curve hold true?
- During which years does the short-term Phillips curve seem to “shift”?
- What could explain the fact that for some years the short-term Phillips curve slopes upward?
- Based on the visual examination of your plot, what is the natural rate of unemployment?